What is a data lake and why should you care?
Knowledge
Aug 3, 2021
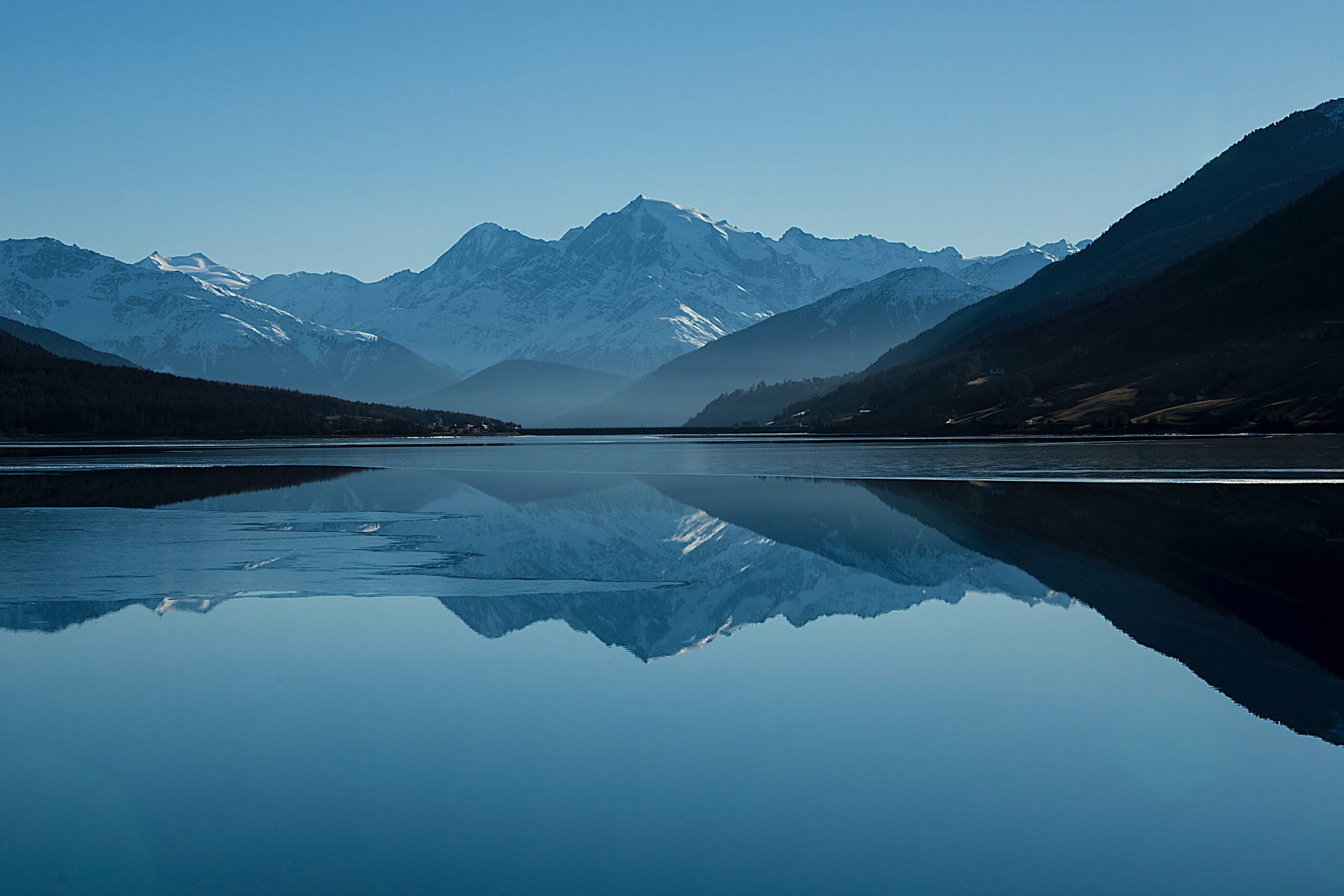
To explain what a data lake and its purpose is, we have to take a step back and consider where we come from. Most modern IT businesses have developed sophisticated databases to hold their crucial data. That may be customer data, order data and so on. Historically these systems have been designed to serve their business needs, in particular
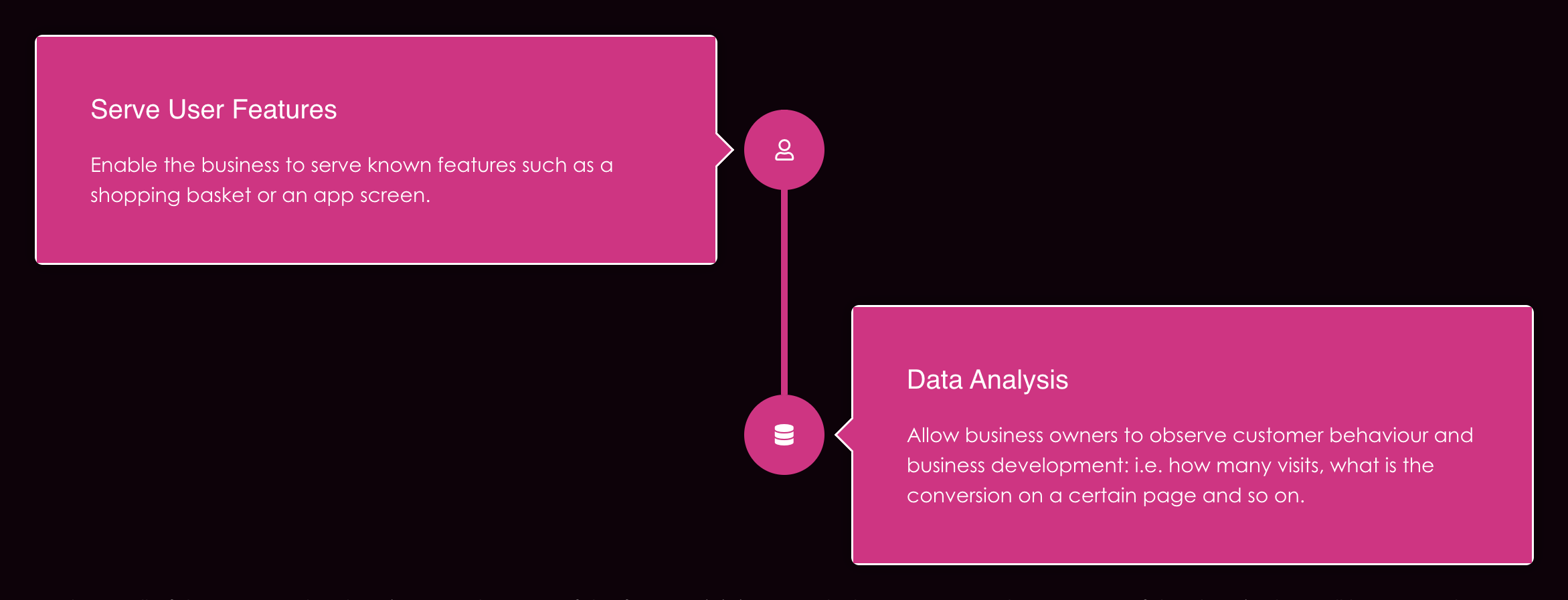
In almost all of these cases the data is not at the core of the feature, it is just needed. In most cases the structure of the data is also well known and changes rarely. If anything, adding a new field – or worse, removing one – can be a hazardous operation that ends up breaking the system. Engineers have been prioritizing schema enforcement and stability, to protect themselves from these issues. And there is no judgment in that, in fact these systems are business critical and deserve rigorous enforcement and a focus on quality and stability.
But then 2021 happened...
Within the last 5-10 years however, this mindset has changed drastically. Nowadays we can build pure data products that can only exist through our ability to learn from data: Suggesting the perfect hotel. Building a personalized playlist. A medical health assessment. The list could go on and on of course. But it’s not only our use cases that have changed, so has our data. Historically we have largely worked with tabular – database style – data. Nowadays we have verbal customer feedback, written free text in a product review. We have heatmaps of eye gaze that express attention. Images of facial expressions. And we have so much more of it as well. Many of our customers produce terabytes of data in sensors alone, every single day. This is why the requirements for data architecture have changed and why data lakes as the go to architecture for data centric businesses have emerged.
So, without further ado – here’s our little Cheat Sheet on what makes a Date Lake and what a Data Warehouse. As per usual with anything on the internet, this is based on our opinion, our findings across multiple customers. Your impressions may vary and we’d love to discuss them, so feel free to engage below this post.
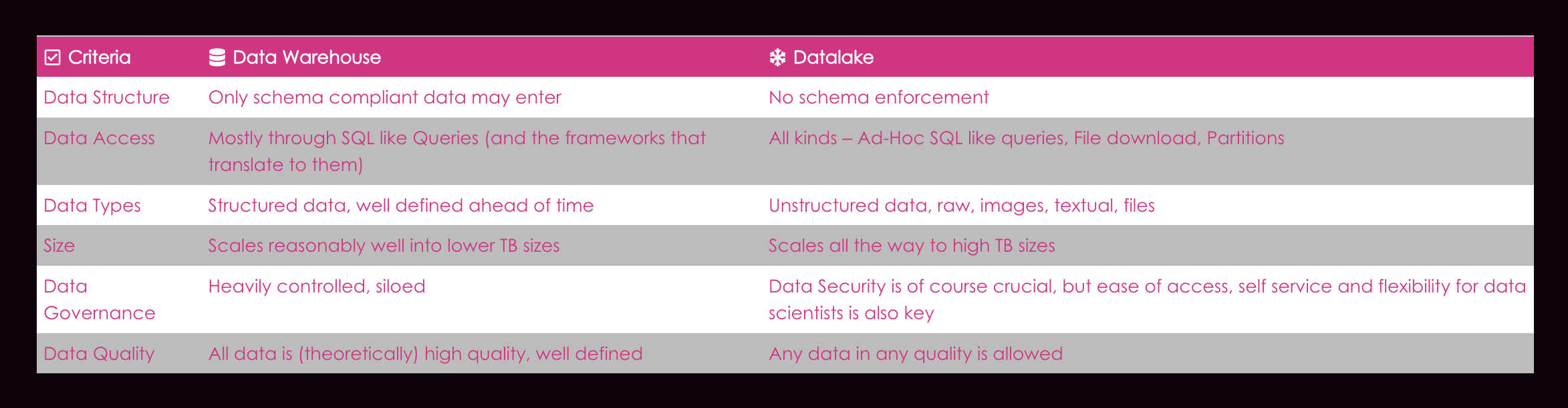
Well then - Should you care?
It depends on where you are in the data journey. Are you collecting and using data to primarily serve your existing customers and features? Or are you already building products that are born from data? That revolve around personalization and machine learning? If you aren’t sure, we can help you find out!
The struggles that most companies are facing these days, no matter how far along in this journey they might be are
- Where will the crucial data come from? Internal apps, the social graph or other, external sources?
- What will constitute the key data to realize future use cases? Documents, customer behaviour? Written messages, containing emotion regarding services or products?
- In other words: what is the data that we should have started collecting today?
“The problem is that, in the world of big data, we don’t really know what value the data has… We might know some questions we want to answer, but not to the extent that it makes sense to close off the ability to answer questions that materialize later.”
Dan Woods in Forbes, 2011
A data lake can help to manage this degree of uncertainty and enable a company to successfully build data products on top of unstructured data. Crucially data lakes are also much better tailored to exploratory, data science work. A data lake should for example enable data scientists to quickly correlate written customer feedback with behaviour from the app for a given timeframe. Lastly data lakes cater to the fact that nobody really knows what will constitute valuable data in the future, no matter which vertical. In a data lake environment, data would rather be stored even if there is no immediate need for it. That can be achieved easily, because nowadays storage is extremely cheap and since we aren’t forced to adhere to governed schemas, we can just store the data as it is, even if it’s quality and purpose – for now – may be debatable.
Weitere Blogs
Kontaktieren Sie uns
Verwirklichen Sie jetzt Ihre KI-Pläne
Wir freuen uns darauf Sie in einem unverbindlichen Gespräch kennenzulernen. Nehmen Sie jetzt mit uns Kontakt auf und wir melden uns umgehend bei Ihnen.
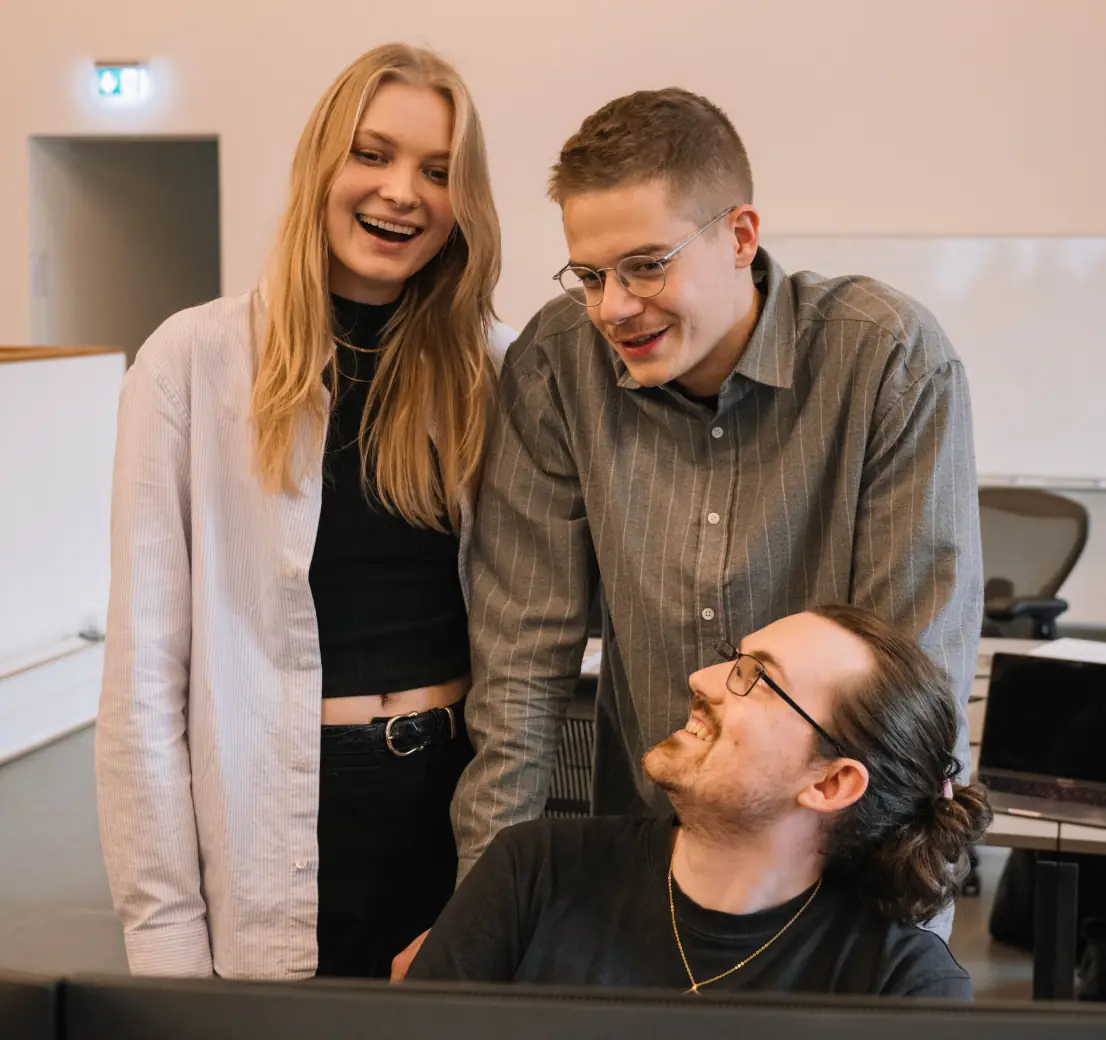
Ihre Anfragepar
Wir werden uns so schnell wie möglich bei Ihnen melden.
Werfen Sie in der Zwischenzeit einen Blick auf die anderen Seiten.